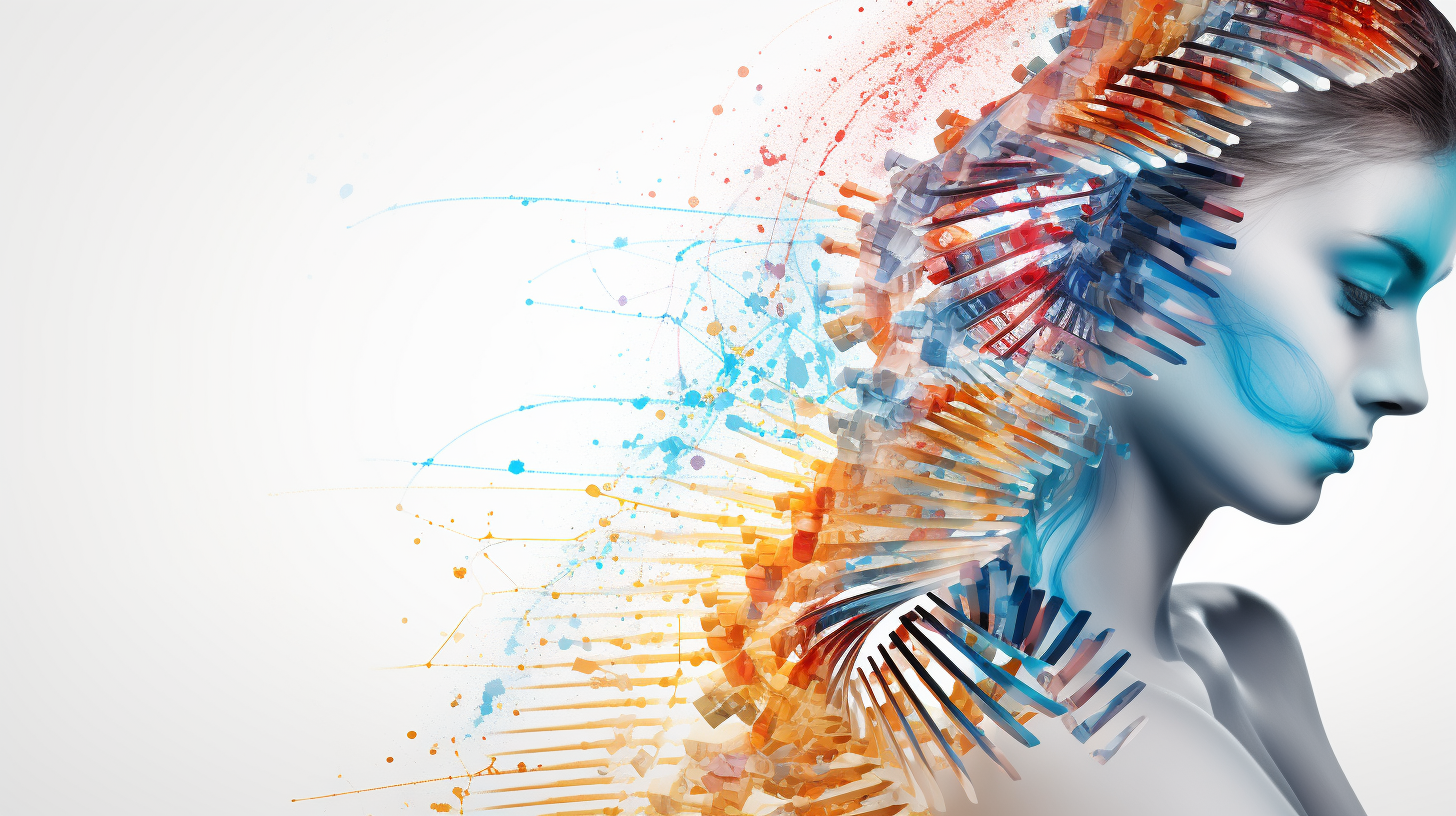
Our Technology
We improve cancer classification by
combining epigenetics with artificial intelligence
The cancer epigenome is a combination of two things: changes in the DNA that happen as the tumor grows, and specific characteristics that show where the cancer started from. This interesting feature allows us to track and find the original source of a specific cancer, even when it has spread to other parts of the body. At Heidelberg Epignostix, we analyze the cancer epigenome to precisely characterize the past and present state of the cancer cells – giving us a molecular fingerprint of the tumor’s life history.
We use a random forest-based model to classify tumors. Random forest is a powerful machine learning / artificial intelligence algorithm used for classification tasks. It works by creating multiple decision trees and combining their predictions to make more accurate and robust classifications. Random forests can analyze the unique methylation patterns in tumor samples and use this information to distinguish different types of tumors. By training the algorithm on a dataset with known tumor subtypes, it can learn to identify specific methylation patterns associated with each tumor type. Once trained, the random forest model can classify new tumor samples based on their methylation profiles, providing valuable insights into tumor biology and behavior.
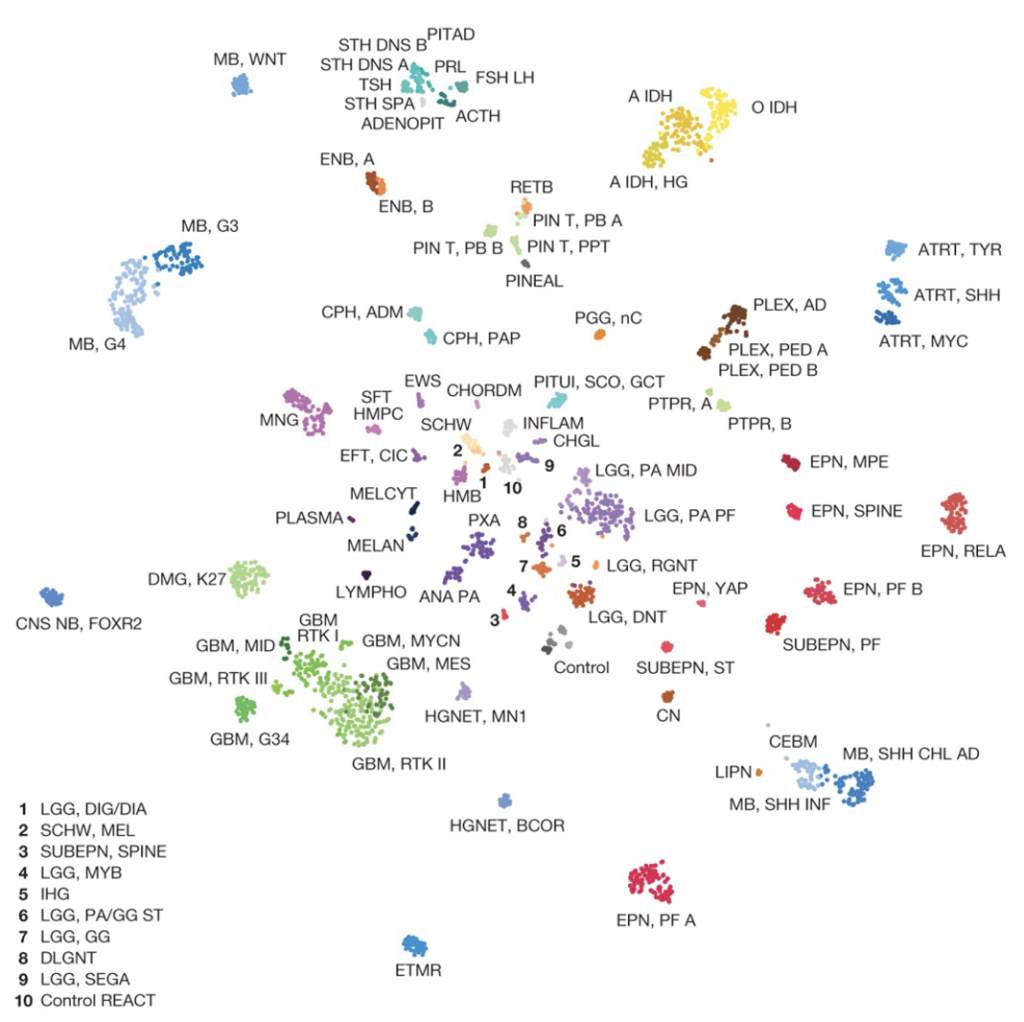
In our breakthrough paper published in Nature 2018, we demonstrated the first methylation-based classification of central nervous system tumors.
The approach complements, extends and, in some cases, supersedes the tumour-diagnostic potential of microscopic examination.
News and Views (Nature) on the Capper et. al 2018 article describing the methylation classifier
Capper et. al 2018 Nature
Our products
We develop innovative solutions that harness the power of DNA methylation analysis to revolutionize cancer classification.
*All are classifiers are for Research Use Only
Heidelberg Brain Tumour Classifier
The Heidelberg Brain Tumour Classifier now covers over 150 types of CNS tumors. Established and distributed under the Molecular Neuropathology (MNP) banner, the classifier has been widely used since 2017. To-date the web portal has had >120,000 uploads from across the world. Our flagship product is now exclusively available on our website.
*To implement the classifier you are required to generate and upload unprocessed IDAT-files of Illumina Human Methylation 450 BeadChip arrays or EPIC v1/v2 BeadChip arrays of your samples of interest. Within a short time you will receive a report of the methylation profiling of your case, a copy number plot and an estimation of MGMT promoter methylation status.
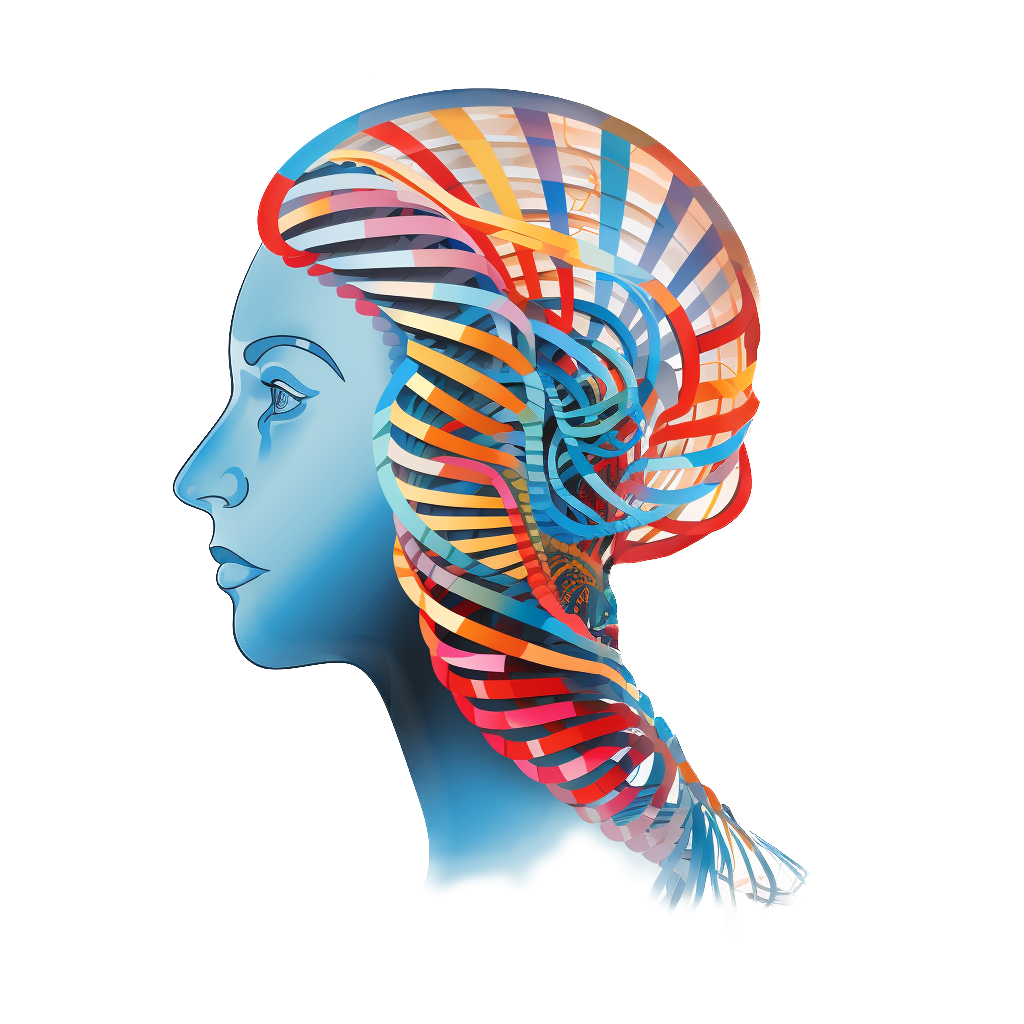
All our products are available for free for academic research use.
In the pipeline
We are actively working on a range of classifiers designed to address different cancer types. Our team of experts is committed to leveraging DNA methylation patterns to unlock valuable insights into tumor biology and progression. These classifiers are under development and aim to offer tailored treatment strategies and improved patient outcomes for various cancers in the near future.
We understand that each clinical set-up presents unique challenges, and we are dedicated to adopting state-of-the-art tools and methodologies to improve access to precision diagnostics. From third generation sequencing-based beta versions to other cutting-edge approaches, we are constantly pushing the boundaries to deliver the most advanced classifiers in the field.
Sarcoma classifier
Our advanced sarcoma classifier
- Early version published in Nature Communications
- Now includes >60 sarcoma classes
- More data and refined classes
- Includes relevant clinical annotation of class characteristics
More classifiers
A range of new classifiers covering a variety of cancers
- Multiple classifiers for different cancer types
- Early versions include epithelial cancers like skin cancer and many more
- Classes curated by expert pathologists
Nanopore classifier
Our classifiers now also work with Nanopore sequencing methylation calls
- Adopts state-of-the art technologies
- Methylation calls from portable Nanopore sequencers
- No ad-hoc models needed – one consistent classifier
- Includes all available classifiers